Cursos

Modelado de problemas típicos en su industria
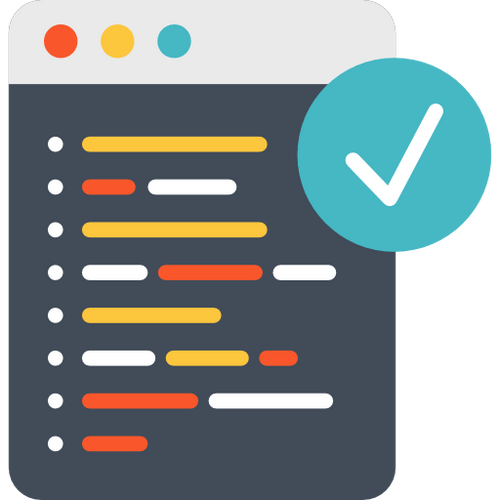
Programación Entera Mixta (MLP) o Constranint Programing (COP)
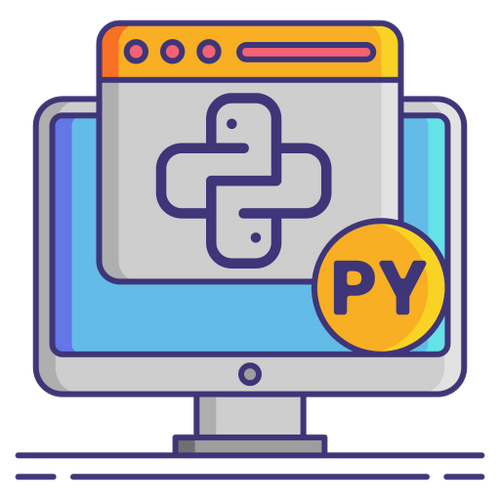
Uso del lenguaje OPL o APIs programadas en (C. C++, Java, Python)
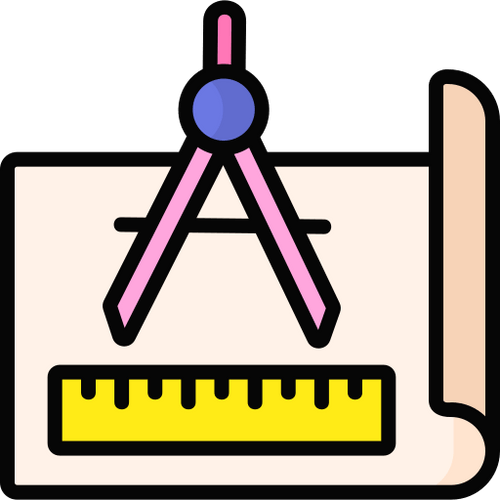
Técnicas avanzadas (generación de columnas, descomposición de Bender)
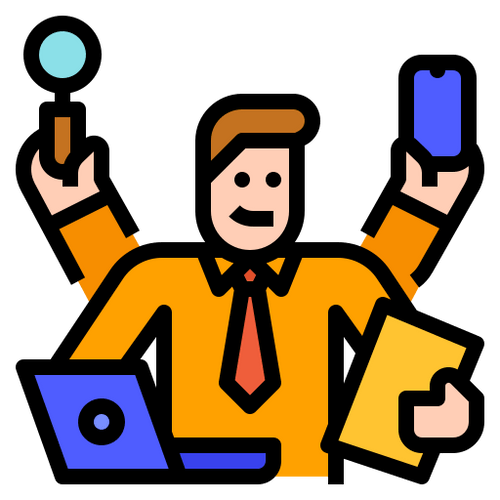